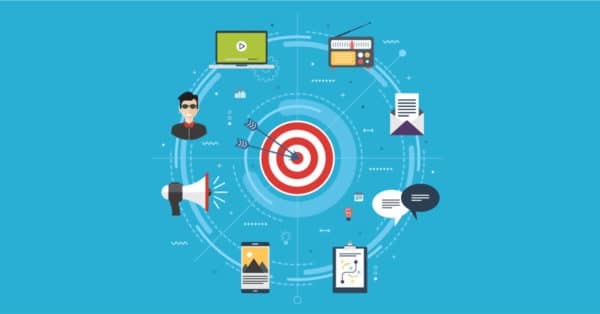
Marketing mix modeling is one of the most powerful and sophisticated tools for measuring marketing’s impact on business goals. And it can be intimidating, especially to marketers who don’t have much background in data science.
But at a high level, it’s pretty easy to understand.
It only takes three things to build a marketing mix model:
- You need to know how much you spent in each marketing channel, both online and offline. Ideally, you should know the level of spend by day for at least 18 months.
- You need to know how much awareness each of those channels created in that time period.
- You need to know your target (or dependent) variable. It’s what you’re trying to accomplish with your marketing campaigns. That might be increased revenue, market share, leads or even website sessions.
If you have those three elements — and your data is clean and well-structured — you can then apply a regression model to determine each channel’s contribution to your desired result.
This is a simplified example, but the better models will even look at performance when spending in both channels simultaneously:
“If I spend $1 on TV ads, it usually leads to $3 in revenue. For every $1 spent on search ads, we see $2 in revenue. And if we spend $1 on each channel at the same time, we have an even greater combined return of $7.”
And that’s valuable information! Using “what if” scenario planning, you can estimate how much you should spend and where in order to hit your next campaign’s goals. (We’ve built a marketing mix calculator that lets our clients do that.)
Other Factors in Marketing Mix Modeling
The three elements mentioned above are the absolute basics of a marketing mix model. A big benefit of marketing mix modeling is that you can incorporate other real-world variables into your analysis.
One of the biggest is seasonality. If you’re a retailer, then Christmas probably has a big impact on your sales. You can design your model to account for that annual bump.
Or maybe you need to consider weather in your model because you sell something (like car batteries or propane tanks) with sales that change with the temperature.
Seasonality and weather are where we usually start with MMM, but your marketing mix model can account for a wealth of other factors such as pricing, macroeconomic conditions, competition and specific promotions, to name just a few.
Most models also try to distinguish between base and incremental sales.
- Base sales are how much you would sell if you didn’t do any marketing at all. They’re affected by long-term, relatively stable factors like pricing, distribution and brand reputation.
- Incremental sales are the result of marketing “doing stuff” — buying media and running promotions.
Marketing Mix Modeling vs. Marketing Attribution
Sometimes people confuse marketing mix modeling with marketing attribution because they’re both used to determine marketing’s impact on business goals.
They just come at this problem from two different directions.
Marketing Mix Models Are Top-Down
With MMM, you need to understand how much you spent, where you spent it, and what happened as a result. But you don’t have to know anything about the customer journey or how an individual interacted with your campaigns.
Did Bob see a search ad before he bought a can of soup? Was it a TV commercial? Who cares! You’re just dealing with aggregate data.
In fact, CPG brands were one of the first users of marketing mix modeling because they didn’t have any of that customer-level data.
Marketing Attribution Is Bottom-Up
Attribution looks at user-level interactions to determine what specific campaigns, channels and tactics led to a desired result.
Let’s say you want to see if your Facebook ad led to people visiting your website, either as a view-through or click-through conversion. At some level, you’re tracking an individual’s clicks to see if the same person who saw your ad went to your site.
Or maybe you use Google Analytics to determine where your website visitors are coming from and what they’re doing on your site. That path data — even when viewed in the aggregate, anonymously — is generated by tracking specific users.
Ditto for data-driven attribution, which combines click and path data with media spend data.
Attribution is useful for quickly optimizing campaign tactics, because you can see which channels or messages are leading people to convert.
But what if you can’t track specific users? That’s becoming harder and harder, partly because of regulations like GDPR and CCPA, and partly because the big tech companies are moving away from third-party cookies that help measure individual actions.
This is why marketing mix modeling is so important. It gives you that big-picture view of marketing ROI across all channels without requiring you to track every single marketing touchpoint.
Meanwhile, attribution — even if you can’t track all individuals — still gives you directionally useful insight on how tactics can be optimized.
If possible, marketing teams should use MMM and attribution to get a more complete picture of performance.
Assuming, of course, that marketing mix modeling makes sense for you.
BLOG: The Marketer’s Quick-Start Guide to Markov Chains
The Limitations of Marketing Mix Models
Marketing mix modeling isn’t the right choice for every marketing team. Before you use MMM, you should be aware of a few potential drawbacks:
- A marketing mix model shows correlation, not causation. Your model might indicate that sales increased 10 percent when you had TV ads in market, but you can’t actually prove your TV ads were responsible for the sales bump.
- Marketing mix modeling, as a rule of thumb, should be used when you’re spending at least $2 million annually on media spend.
- A marketing mix model is better for planning upcoming campaigns. It just takes too long to use MMM to optimize campaigns that are currently running. You would get faster results with an attribution model.
- Marketing mix models aren’t great for planning campaigns for new products. Your historical data might not be relevant to a new product.
- Marketing mix models also may not be as useful for measuring marketing’s impact on long-term brand equity, which can take longer to respond to marketing investment.
- If something dramatic or unexpected happens, like a natural disaster in your target markets, your model might no longer be accurate because it’s based on historical data.
Worth the Investment
This is a high-level look at marketing mix modeling. You now know how it works in concept, how it can help your marketing efforts and when it should (or shouldn’t) be used.
Actually building and using a marketing mix model will be more challenging. You’ll need at least 18 months of clean, well-structured data and the help of a data scientist who can help you develop a model tailored to your unique situation.
But the benefits of MMM are worth the investment, not just for understanding your performance so far, but for planning more effective campaigns going forward.
Harness the Power of Predictive Analytics
Alight’s end-to-end solutions bring marketing mix modeling to any marketing team without requiring advanced data science skills. Want to learn how it all works? Schedule a call with our team!